The data flood is here, beware of drowning.
Airports gain more and more access to a variety of data sources. This evolution requires to investigate the analytic opportunities that these data sets might offer. But, more data doesn´t mean automatically more value. All obtained data has to be qualified, transformed and turned into a valuable asset. At the end of the day the major question remains always the same: How to gain value with the data we have?
Where does that data come from?
First, let´s have a look where that data is coming from. During the late 90´s, it was obvious that a technical revolution will take place which will affect the Aviation branch as well. Still working with tons of paper work, computer systems changed the way of passenger and aircraft handling. Gaining speed and reliability during daily operations was the effect. Beside this, the produced data could be stored in a much more efficient way. Over time, the benefits for reporting, analytics and research was identified. Nevertheless, especially within the Terminal environment, creating the right measures was long time a complicated thing. Manual measurements and transformation of paper reports into data sheets were inefficient and no continuous tasks. Then finally, the first automatic detection technologies to count passenger flows were launched about 10 years ago. In addition, the seamless passenger journey began to rise and the use of self-service counters for check-in, bag drop and boarding as well as biometric technology created additional insights into passenger behaviour. Putting all these strings together sounds logical but needs a conceptual approach.
More data doesn´t mean automatically more intelligence. You have to make data smart.
How to start with data analytics?
Big data was one of the big phrases the IT industry hyped within the last couple of years. In fact, this just meant how to store huge amount of data and how to process data queries as quick as possible. The industry tried to sell these technologies with the huge capabilities of BI. To be honest, BI doesn´t solve the problem itself. It creates the basis to get to a potential solution. There are still analytic capabilties needed to turn big data into smart data. Therefore, I want focus in the following on four major analytic methodologies. These methodologies must not be seen as competitive approaches, but much more as complement to each other and the foundation of the real power of business intelligence:
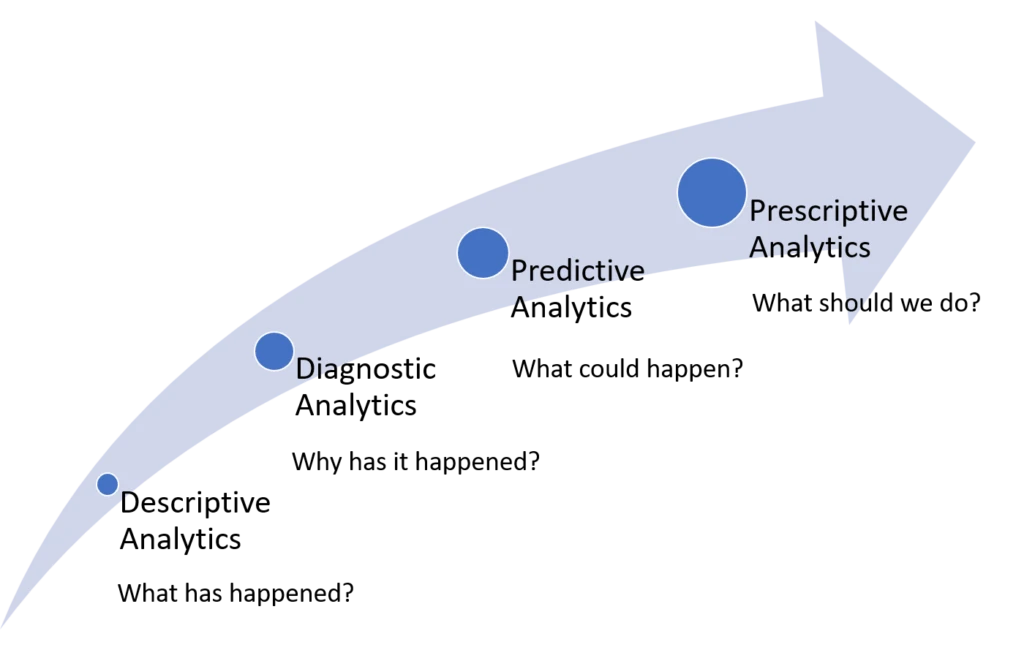
- Descriptive Analytics, which use data aggregation and data mining to provide insight into the past and answer: “What has happened?”
- Diagnostic Analytics, which also use data mining to create root-cause analysis about past events and answer: “Why has it happened?”
- Predictive Analytics, which use statistical models and forecasts techniques to understand the future and answer: “What could happen?”
- Prescriptive Analytics, which use optimization and simulation algorithms to advice on possible outcomes and answer: “What should we do?
Descriptive Analytics: Understanding the past
Descriptive analysis or statistics does exactly what the name implies they describe or summarize raw data and make it something that is interpretable. They are analytics that describe the past, which can refer to any point of time that an event has occurred, whether it is one minute ago, or one year ago. Descriptive analytics are useful because they allow us to learn from past behaviours, and understand how they might influence future outcomes.
Let´s look at an example from the airport industry to make it more grabable. A planner wants to optimize the process at a check-point. The first step would be to analyse the process and data sets available. Flight-, Passenger- and Check-point related information will give him insights about the process to determine the relevant KPI´s. This process of data mining is very crucial as a first step.
Diagnostic Analytics: Understanding the causes
Diagnostic Analytics can be seen as contemplary approach to the Descriptive step, beside describing the past, to understand the effects and process varieties that were responsible for the data created. Deviances or exceptions can be statistically excluded when creating descriptive models, but in order to understand, they need to be further analysed.
Looking at the topic of check-point optimization this would mean, it is not enough to describe a KPI, it is also necessary to be able to explain why a certain value differs from time to time. The capabilities to determine certain conditions will be helpful in the later stage of future scenario evaluation.
Predictive Analytics: Transforming into the future
Predictive analytics has its roots in the ability to look into the future what might happen. These analytics are about understanding the future. Predictive analytics provides companies with actionable insights based on data. Predictive analytics provide estimates about the likelihood of a future outcome. It is important to remember that no statistical algorithm can “predict” the future with 100% certainty. This is because the foundation of predictive analytics is based on probabilities.
Coming back to the example of security check-points, by the description of the parameters it is possible to set-up statistic models to forecast for instance the passenger demand at a certain check-point. Transforming this into future trends this intends to show the business owner how his process is going to perform based on the current rule definitions.
Prescriptive Analytics: Creating advise
The relatively new field of prescriptive analytics allows users to define a number of different possible actions and guide them towards a solution. In a nut-shell, these analytics are all about providing advice. Prescriptive analytics attempt to quantify the effect of future decisions in order to advise on possible outcomes before the decisions are actually made. At their best, prescriptive analytics predicts not only what will happen, but also why it will happen providing recommendations regarding actions that will take advantage of the predictions.
Now, there comes the final stage of analysis for our check-point planner. By using simulation techniques and turning the output of data description and prediction into action, he will gain insights about different scenarios and set-ups. This evaluation is extremely helpful, when considering future changes to face with potential actions. These changes could happen in the Terminal layout, process changes or limitation of resources. Potential actions could be re-allocation of flights, floor space or resource adjustments. It is necessary to create those different scenarios to be able to judge and decide for the best action to take to react according to these changes.
All together these analytic approaches create a powerful concept to bring your business to the next stage.
How can Machine Learning help ?
In the beginning of BI, all these analytic steps described above required intensive work, technical and analytic skills as well as lots of time to perform these. Data scientists had to have mathematical skills as well as IT knowledge about software, SQL queries or even specific programming language like Base. But, the technical evolution helped users to get faster into this topic and their hands on their own analytics. Recently, we just entered a new era of data analytics. The key-words is: Machine Learning. Machine Learning algorithms are based on complex mathematics and statistical formulas to automize the process of setting up predictive rule sets. The positive thing about machine learning is, if built up correctly, it is true end-user support without being that black box no one understands.
Machine Learning improves the speed of setting up rules without being a black box
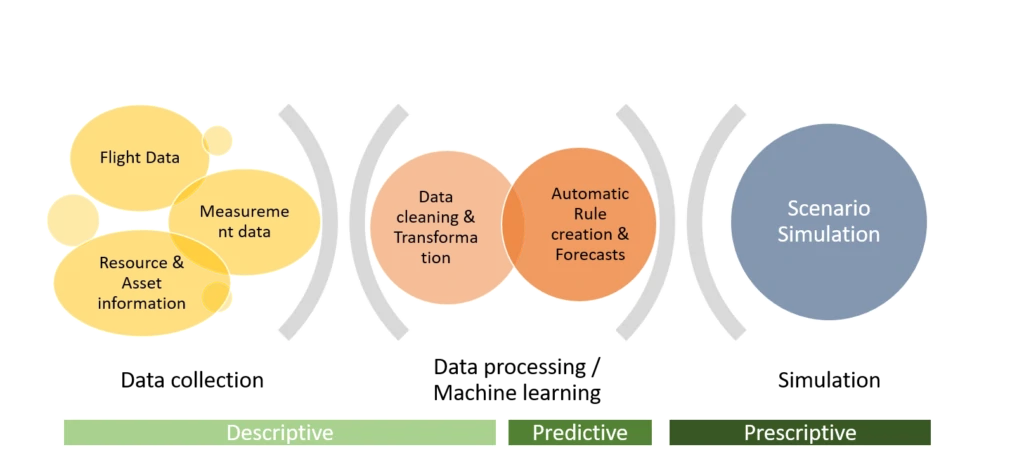
Amorph Systems, an expert company in providing simulation software to the airport industry, focused on the advantages of integrating Machine Learning into its AMORPH.aero platform to optimize end user capabilities. This created a unique and industry ahead platform for Nextgen predictions with a combination of best available techniques.
If you want to learn more, please contact us at info@amorph.com